Think Tank
Cognitive managed services across telco operations and what it takes to be truly data-driven enterprise – The new mantra for operational transformation
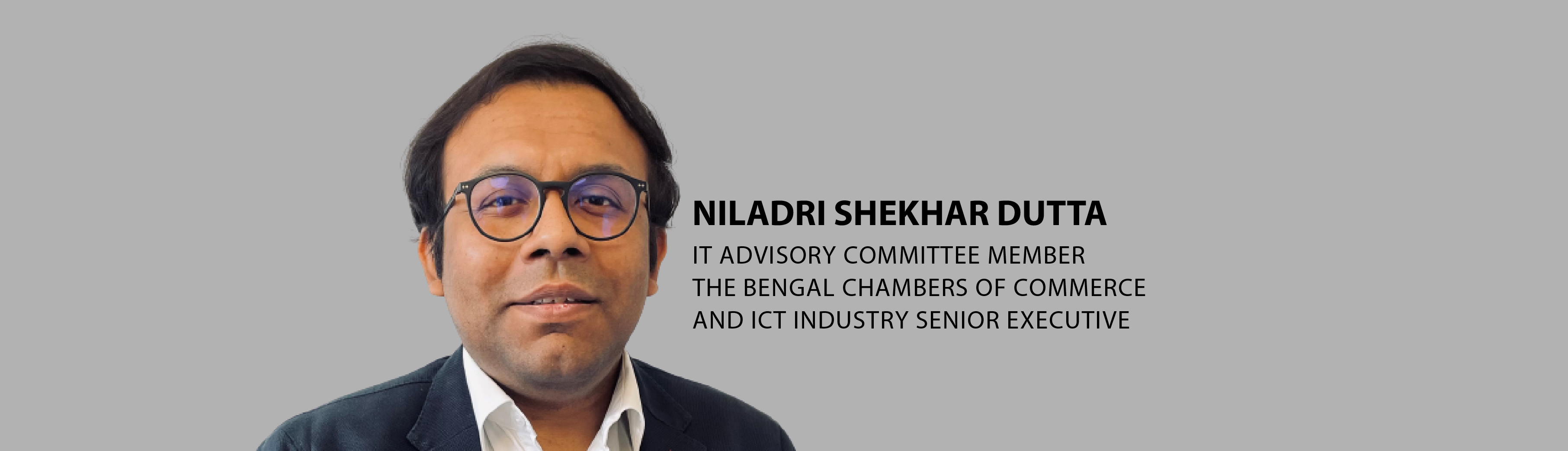
Telcos of the modern era are looking to differentiate themselves with AI and data-driven use cases in their business as well as operations. They are mostly doing so to demonstrate the tangible value of technologies and platforms, which they have invested in, to drive key transformation changes within their set up due to the advent of digitization and business model changes. It is imperative for telcos to drive operational transformation in this new paradigm and relook at the legacy operating models that they have been driving all these years to ensure this convergence of networks and IT becomes seamless. With the advent of 5G, IoT, and cloud technologies, telcos are soon becoming service enablers and the new eco-system demands greater complexity of business services and increased automation around it. Hence, the need is to get hold of the real-time or near real-time data points to manage this effectively and efficiently as much as possible. We all know data is the new oil, hence, to drive this as a differentiator and ensure the dedicated operational intelligence prevails one needs to use data as the lowest common denominator and a single instance of truth in their operations. To do this, it is imperative to understand the context of the data, which we often term as the meta-data followed by the complexity of the associated business and operational processes supported and the underlying architecture landscape. Today, every telecom operator most definitely does have the ambition to be data-driven, but very few know the means to achieve it and, therefore, look for best implementation approaches. Most of these concepts stick around only at the design level and do not travel the distance beyond it. We must look for avenues to ensure this data-driven operational implementation is real, and is fully traced back to the origin of the core business services, which are being supported by the complex services portfolio that the telco is offering, backed by the core business and operational processes and implemented by specific data and AI use cases. It is then our priority to manage this list of AI and data use cases with proper involvement of automation to ensure the real impactful messages are being delivered across CxOs, which will eventually lead to either being customer-centric or being operationally super-efficient, driving revenue or profitability. One must not forget that every CIO or IT/technology function is eventually viewed as a cost center in any telecom operator, thus influencing the very aspect of revenue or cost with tangible data points is eventually a dream come true for a CIO. Data-driven operations must empower the CIO or CDO (chief digital/data officer) for any telco to make effective choices of investments and help in strategy formulation and execution in the long run to drive the operator’s business and operational agenda.
More often than not, managed services across networks and IT function is an outsourcing decision, which commonly lies with the CTO and CIO, who eventually, looking at economies of scale, lines up the most economical deal with the SI or services partner. There is surely a demand for differentiated operations without being too tactical about it. Most outsourcing partners here showcase an efficiency game by picking up TAT (turnaround times) or percent FTR (first time right) improvements without really looking at any strategic KPIs around CSAT (customer satisfaction scores only in case of contact center operations) or NPS (net promoter score) unless asked for while correlating S-KPIs (service KPIs) as per contractual obligations. This is where the game changer in delivering cognitive managed services come into light, where service delivery accounts for something beyond efficiency and can be proved with the examples of data artifacts, which prevail within the operational gambit.
This is often delivered by vanilla automation, now termed as RPA or RBA, to enhance the outcomes of the contractual performance indicators or by amalgamation of the analytics or AI. The underlying fact needs to be that decision making must be driven and empowered by data, which means data models, structures, and overall data fabric and not just by fancy charts and graphs in a dashboard with drill-down capabilities. The question now that really tickles the mind of operational stakeholders is how this will be possible where decision making needs to be mapped into certain key data artifacts, which drives overall operational or business process performance. Most telcos will struggle in this because of two fundamental reasons, the implementation of any solution in the network or IT domain is largely use case driven as depicted by the high level of design scope and the requirements metrics, which is defined during implementation, most definitely do not take into consideration the fact as to what will happen post implementation during operations and managed service, assuming it will be a status-quo, which will be taken care of only when we arrive at the juncture.
In this article, I will propose two different perspectives of how this risk potentially can be mitigated, thereby making cognitive decision making a most organic and natural fitment in the overall setup, especially in IT (OSS-BSS) followed by network software-driven managed services. While implementing any solution, there is a fundamental need to define a data traceability matrix in line with all requirements as addressed by the requirements and there corresponding mapping into solution use cases. This is usually not the case during any implementation as data layer is more often not linked to either application or the business processes under scope of IT or networks. This is a very common error, which most implementations follow as the only aim during the overall life cycle is to focus on the selected use cases so that go-live can happen within the requisite timelines. The need for data traceability helps to understand and track all data sets within multiple applications, and thereby easily helps to define their lineage without any specific pain. Not only this, one can also understand the touch points where quality of data is most vulnerable, given the multi-vendor COTS environment, which often exists. When the same goes to operations driven by managed services, it is very easy to apply any statistical modelling technique in this data workflow, highlighting the data lineages to easily detect exceptions and thereby take corrective actions to resolve them as well. Deployment of LLMs (large language models) also becomes very easy on top of these data sets, which are often termed as CDE – critical data elements.
The other proposed solution will be to identify all possible business rules, which are within the applied processes in scope and then filter out the exact data sets, which dictate the performance of these rules, which thereby has an impact on the core process itself. This concept can largely be extrapolated as process-data integration or synergy driving process and data. The key thing is the convergence and the seamless experience of process and data integration, which will eventually help in identifying exceptions by looking at these very data elements or structures and identifying their faults and exceptions. Hence, data quality becomes utmost important as it drives the integrity of data within the desired overall operational setup. Cognitive operations will require implementation of AI algorithms on top of these data sets while assuming their data quality is accurate enough and is handled in an automated fashion.
Both proposed options are very much achievable by changing the process of implementation and managed services or as a prelude must have requisite to any managed services operations. The unfortunate reality is most telcos do not do it, despite acknowledging these benefits. The general tendency is to straightaway implement AI models post RPA and then claim to be pro-active and intelligent. Deployment of AI models on inaccurate data leads to unexpected outcomes, hence the desirable results are not often visible in cognitive operations. The recommendation will be to ensure, and in order to data driven and pro-active, the empowerment needs to be given to elements at the data layer, which drives process efficiency, hence the need of synergizing all processes with critical data sets and also identifying the traceability of these data across the process life cycle to easily note different touch points, which may exist as most vulnerable. Baselining all decision paradigms with data sets is the true data-driven operation, which telcos should aim for and then put the visualization layer as the last mile to improve reporting.
In future, I am confident that such aspects will take precedence and will be seen as an active ingredient in most large-scale managed services. Data quality platforms and tools with the ability to mine data will be the need of the hour for any standard operation, which has the reason and the intent to boast about being data-driven. Hope this becomes a mass phenomenon as operations converge across networks and IT, and differentiated automation becomes a key trend in days to come.
This article is authored by Niladri Shekhar Dutta, BCC & IIT Advisory Committee member & ICT Industry Personnel. Views expressed are personal.
You must be logged in to post a comment Login